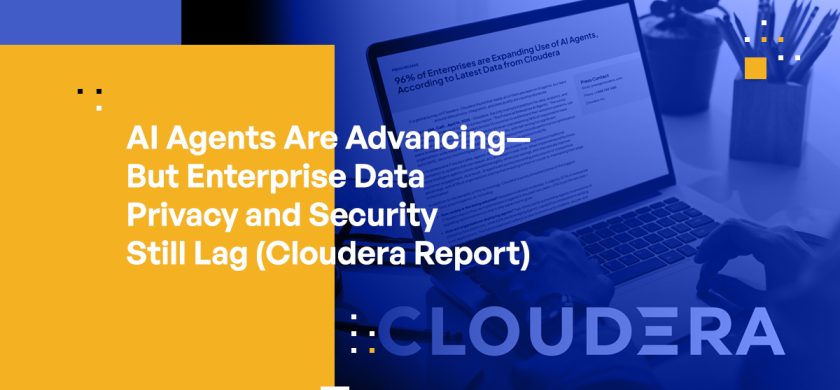
AI Agents Are Advancing—But Enterprise Data Privacy and Security Still Lag (Cloudera Report)
As enterprise interest in AI reaches unprecedented heights, one truth becomes increasingly apparent: trust hasn’t kept pace with adoption. Cloudera’s latest global report, The Future of Enterprise AI Agents, reveals a telling paradox—while an overwhelming 96% of organizations plan to expand their use of AI agents over the next year, more than half identify data privacy as the primary obstacle standing in their way.
This concern isn’t unfounded. AI agents represent far more than mere productivity tools. They function as autonomous systems capable of analyzing data, making complex decisions, and executing multi-step tasks across various enterprise domains—from IT systems and infrastructure to direct customer interactions. With this level of access and independence, the potential risks of data leakage, regulatory violations, and security breaches increase significantly.
In this article, we’ll explore the driving forces behind AI agent adoption, examine why data privacy has emerged as the predominant concern, and discuss practical approaches for organizations to balance innovation with necessary controls. We’ll also consider why simply scaling AI deployment isn’t sufficient—and how solutions like the Kiteworks AI Data Gateway provide a more secure path forward for enterprises committed to responsible AI implementation.
What AI Agents Truly Are—and Their Enterprise Applications
AI agents have rapidly evolved from theoretical concept to practical reality. Unlike conventional chatbots that follow predetermined workflows, these sophisticated systems are designed to reason and act with substantial independence. They can adjust cloud resource allocations in real-time, provide assistance with software development projects, offer recommendations in customer support scenarios, and even contribute insights for financial analysis.
Cloudera’s research indicates that organizations are now integrating these agents into business-critical functions including IT operations management, process automation systems, and predictive analytics platforms. These agents increasingly behave more like digital colleagues than simple tools—they collaborate with human workers, analyze information continuously, and make consequential decisions based on defined goals rather than following rigid, step-by-step instructions.
This capability brings enormous potential for productivity enhancements, particularly in areas such as customer service delivery and operational efficiency. However, this advancement comes with significant tradeoffs. Granting AI agents greater autonomy necessarily means providing them access to more organizational data. In many instances, this data is sensitive, subject to regulatory oversight, or both—creating a tension between innovation and protection that organizations must carefully navigate.
Cloudera’s Report Findings: High Adoption Alongside Significant Risk
In February 2025, Cloudera conducted a comprehensive survey involving nearly 1,500 senior IT leaders across 14 countries. The results paint a clear picture: while AI agent adoption continues accelerating rapidly, serious concerns about data privacy, integration complexity, and regulatory compliance are constraining how quickly—and how extensively—enterprises are willing to deploy these technologies.
According to the report, a majority 53% of organizations identified data privacy as their foremost concern regarding AI agent implementation. This figure surpasses all other potential obstacles, including integration challenges with legacy systems and the substantial costs associated with deployment. For heavily regulated industries such as healthcare and financial services, where compliance requirements are particularly stringent and the consequences of data exposure especially severe, these stakes become even higher.
The report emphasizes that trust, even more than technological capability, represents the determining factor in whether organizations will expand their AI agent deployments. Until companies can establish confidence that AI systems won’t inappropriately access, expose, or misuse sensitive information, those deployments will remain limited in scope—or perhaps shelved entirely.
Where True Risk Resides: Data Access, Not Just Model Behavior
Much of the conversation surrounding AI security has centered on model-specific concerns—avoiding hallucinations, minimizing bias, and preventing adversarial prompting. However, for enterprise security leaders, the more immediate concern involves data access patterns. AI agents must extract information from multiple interconnected systems to perform their intended functions. Without carefully designed guardrails, this necessity creates substantial organizational risk.
When an AI agent retrieves customer information to assist a service representative or accesses operational data to automate IT processes, it must do so within clearly defined boundaries. Unfortunately, these boundaries often remain undefined or poorly enforced. AI agents can potentially access files, databases, and communication threads without clear limitations. This creates opportunities for unauthorized data exposure, non-compliant handling of protected information, and even inadvertent transfers of proprietary intellectual assets to external systems.
The potential failure scenarios aren’t difficult to envision. In highly regulated environments where data access must be meticulously controlled and documented, traditional monitoring solutions weren’t designed to track AI agent activities—much less predict their future actions based on changing conditions or new instructions.
Data Privacy, Compliance Requirements, and Control Mechanisms
The widening gap between AI capabilities and enterprise data governance structures poses increasing challenges. Regulations including GDPR, HIPAA, and the California Consumer Privacy Act require organizations to maintain strict control over personal data usage and processing. These regulatory frameworks weren’t designed with autonomous systems in mind—particularly those capable of independent action across multiple computing environments and data repositories.
Cloudera’s report highlights how this fundamental mismatch affects enterprise confidence in AI deployment. Within many organizations, legal and compliance teams increasingly request implementation delays—not because they fundamentally oppose AI adoption, but because they cannot verify that AI agents will operate consistently within existing governance frameworks. The result is growing demand for data-centric security strategies that align technological innovation with regulatory requirements.
Solutions like the Kiteworks AI Data Gateway help address this gap by creating a secure intermediate layer that governs what data AI agents can access, log, and process. This approach gives organizations the visibility and policy enforcement capabilities they need to deploy AI confidently. Rather than blindly trusting AI tools, enterprises can ensure every interaction with sensitive content remains tracked, managed, and compliant with both corporate policies and external regulations.
Building a Responsible Implementation Approach
Adopting AI agents responsibly doesn’t necessarily mean slowing innovation. Instead, it means beginning with appropriate foundations. Many organizations find success by first deploying AI agents in lower-risk contexts, such as internal IT support functions or non-customer-facing operational workflows. These initial deployments allow companies to observe agent behaviors, understand data flow patterns, and identify potential governance gaps—all without placing sensitive information at significant risk.
Once trust becomes established through these controlled implementations, scaling becomes considerably easier. However, expansion also requires reconsidering how responsibility gets assigned within the organization. AI agents don’t simply present information for human consideration; they actively take consequential actions. This means organizations must clearly determine accountability when an agent makes decisions—whether that responsibility falls to development teams, data stewards, or the business units implementing the system.
Transparency represents another essential element. Enterprises must maintain the ability to audit decision-making processes, track which data sources were accessed for specific operations, and verify whether agent actions align with established enterprise policies. This oversight becomes difficult to achieve without specialized systems designed specifically to monitor and control AI behavior at the data level.
Lessons from Documented Implementation Failures
Cloudera’s report identifies several sectors where the risks associated with AI deployment have already materialized in concerning ways. In healthcare settings, diagnostic agents trained on non-representative data have generated inaccurate recommendations that disproportionately impact underrepresented demographic groups. In defense applications, biased AI decision-making has raised profound ethical questions regarding machine involvement in high-stakes operational environments.
These aren’t theoretical concerns but practical reminders that data quality, procedural transparency, and effective control mechanisms remain essential for AI systems to earn—and maintain—organizational trust. When AI systems operate without appropriate visibility or oversight, the consequences extend beyond technical considerations to impact real people and organizations. This reality underscores why responsible deployment practices must develop alongside robust data governance capabilities.
The Human Factor in AI Implementation
Beyond technical considerations, successful AI agent deployment depends heavily on human factors. Organizations must invest in training programs that help employees understand how to work effectively alongside increasingly autonomous systems. This includes developing skills for appropriate task delegation, interpreting AI recommendations critically, and maintaining awareness of situations where human judgment should override automated suggestions.
The organizations achieving the greatest success with AI agents aren’t necessarily those with the most sophisticated models or largest data repositories. Rather, they’re the ones that have thoughtfully considered the human-machine partnership, establishing clear protocols for collaboration while maintaining human oversight of critical decisions. This balanced approach recognizes that AI systems, despite their remarkable capabilities, still lack the contextual understanding and ethical judgment that human workers bring to complex situations.
Effective implementation also requires cross-functional alignment. Technical teams responsible for AI development must collaborate closely with business units, compliance specialists, and risk management professionals. This collaborative approach ensures that AI agents receive proper guidance from diverse perspectives rather than optimizing for narrow technical objectives that might conflict with broader organizational priorities.
Balancing Innovation and Protection
Perhaps the most challenging aspect of enterprise AI implementation involves striking the right balance between enabling innovation and maintaining appropriate safeguards. Too many restrictions can stifle AI’s transformative potential, while insufficient controls create unacceptable risks. Finding this equilibrium requires ongoing dialogue between technology enthusiasts and those responsible for organizational protection.
Successful organizations approach this challenge pragmatically. Rather than viewing security and compliance requirements as obstacles to innovation, they recognize these considerations as essential components of sustainable AI deployment. By incorporating governance principles early in the development process rather than treating them as afterthoughts, these companies create AI implementations that deliver business value while remaining aligned with organizational values and regulatory expectations.
This integrated approach also creates competitive advantages. Organizations that establish trustworthy AI frameworks can move more confidently into new application areas, knowing their governance foundations will support responsible expansion. Meanwhile, companies that prioritize speed over security often find themselves forced to revisit implementations later—costing more in remediation than they would have spent on proper controls initially.
Building for the Future: AI Governance as Strategic Investment
Forward-thinking enterprises increasingly view robust AI governance not as compliance overhead but as strategic investment. The organizations that establish comprehensive data protection capabilities today position themselves advantageously for tomorrow’s more sophisticated AI applications. As autonomous systems become more deeply integrated with critical business functions, the value of responsible frameworks only increases.
This perspective frames governance not as restricting what AI can do, but as enabling what it should do—creating guardrails that channel innovation toward beneficial outcomes while preventing foreseeable harms. When AI agents operate within clearly defined ethical and operational boundaries, they become more valuable to the organization, not less.
The most advanced organizations have begun developing AI-specific governance committees that bring together technical expertise, business knowledge, legal guidance, and ethical perspectives. These cross-functional groups establish principles, review implementations, and continuously refine governance approaches as technologies evolve. By institutionalizing this collaborative framework, they ensure AI applications receive appropriate scrutiny without creating unnecessary barriers to beneficial innovation.
Conclusion: Trust as Competitive Advantage
AI agents have secured their place in the enterprise technology landscape. Organizations recognize their tremendous value potential and continue investing heavily in expanded implementations. However, adoption without trust creates significant organizational risk. As Cloudera’s research clearly demonstrates, the greatest opportunity—and the most substantial challenge—lies in effectively managing data privacy, security, and compliance considerations while scaling AI capabilities across the enterprise.
The fundamental challenge isn’t impeding AI progress but ensuring that progress occurs securely and responsibly. This requires implementing appropriate controls, establishing clear accountability structures, and guaranteeing that every AI interaction with enterprise data remains governed, documented, and compliant with relevant requirements. With solutions like the Kiteworks AI Data Gateway, organizations need not choose between innovation and control—they can pursue both simultaneously.
The enterprises that recognize this reality—and take decisive action accordingly—will be best positioned to transform AI’s considerable promise into sustainable business value. As autonomous systems become increasingly central to competitive strategy, the organizations that establish trustworthy foundations today will enjoy significant advantages tomorrow. In the emerging AI-enabled business landscape, trust doesn’t merely facilitate technology adoption—it becomes a genuine competitive differentiator.
FAQs
AI agents are autonomous systems that can analyze data, make decisions, and execute complex tasks across enterprise environments without constant human supervision. Unlike traditional AI tools that follow rigid instructions, agents can reason independently, adjust to changing conditions, and collaborate with humans in ways that more closely resemble colleagues than simple automation tools. They represent a significant evolution in enterprise AI capabilities but require greater access to organizational data to function effectively.
Data privacy ranks as the primary concern because AI agents need extensive data access across multiple systems to perform their functions. According to Cloudera’s research, 53% of organizations identified data privacy as their biggest adoption obstacle, outranking both technical integration challenges and implementation costs. This concern is especially pronounced in regulated industries where data breaches can trigger severe financial penalties and reputational damage.
Organizations can achieve this balance by starting with lower-risk deployments that don’t involve sensitive data, establishing clear accountability frameworks, implementing specialized monitoring tools designed for AI systems, and utilizing solutions like secure data gateways that control what information agents can access. The most successful implementations treat governance not as a barrier to innovation but as an enabler of sustainable, responsible AI deployment.
Today’s enterprise AI agents are primarily deployed in IT operations management, process automation, predictive analytics, customer support enhancement, and software development assistance. These applications represent areas where autonomous decision-making delivers significant efficiency gains while still allowing for appropriate human oversight of critical functions. As trust increases, deployment areas continue expanding into more business-critical functions.
Early implementation failures highlight the importance of diverse training data, transparent decision-making processes, comprehensive governance frameworks, and cross-functional collaboration. In healthcare, for example, diagnostic agents trained on non-representative data have produced recommendations that disadvantage certain patient groups. These experiences demonstrate why technical capability alone isn’t sufficient—trustworthy AI requires equal attention to ethical considerations, governance structures, and human oversight mechanisms.
Additional Resources
- Blog Post Kiteworks: Fortifying AI Advancements with Data Security
- Press Release Kiteworks Named Founding Member of NIST Artificial Intelligence Safety Institute Consortium
- Blog Post US Executive Order on Artificial Intelligence Demands Safe, Secure, and Trustworthy Development
- Blog Post A Comprehensive Approach to Enhancing Data Security and Privacy in AI Systems
- Blog Post Building Trust in Generative AI with a Zero Trust Approach